Proactive, not Reactive: Predictive marketing uses data analytics to forecast customer behavior and needs, enabling proactive rather than reactive marketing strategies.
Everyday Use Cases: Common use cases include churn prevention, demand forecasting, predictive lead scoring, and content personalization.
Quality Data is Key: To successfully implement predictive marketing, focus on quality data, regulatory compliance, continuous testing, and robust analytics tools.
Predictive marketing in B2B can lead to some remarkable outcomes. For example, Trend Micro shifted to a predictive analytics-powered ABM campaign and saw a 4x increase in new account engagement.
But predictive marketing isn't without challenges. Marketing leaders often struggle with complex integrations. It can also be challenging to prove ROI to stakeholders. Another barrier is low-quality data.
Even with high-quality datasets, not all of your insights will be actionable: Peter Shafer, VP of Sales and Marketing at The Prosper Group, estimates that "probably about 60% of [data] generates noise and maybe 40% of it really generates something that’s that useful and helpful."
This guide will show you how to cut through the noise and create predictive B2B marketing programs that drive real business outcomes. We'll cover everything from choosing the right tools to building data-driven processes that scale with your business—all focused on helping you transform your marketing organization from reactive to predictive.
What Is B2B Predictive Marketing?
Predictive marketing transforms your historical data into actionable insights about future customer behavior. Because you can use predictive marketing to gauge which marketing strategies are most likely to succeed, it results in more cost-effective campaigns.
Here’s how Amit Bivas, former VP of Global Marketing at Optimove, describes the use of predictive marketing day-to-day during his time at the organization:
In practice, this might mean automatically triggering a personalized offer to enterprise accounts showing high purchase intent, or adjusting your ad spend in real-time based on predicted conversion rates.
Predictive marketing can also help you answer questions like:
- Which accounts are most likely to convert in the next 30 days?
- What's the optimal price point for each market segment?
- Which customers are showing early warning signs of churn?
- Where should we allocate our marketing budget for maximum ROI?
After applying a predictive marketing approach, the end result is marketing decisions driven by data, not hunches—and the ability to get ahead of customer needs instead of just responding to them.
Why Bother With B2B Predictive Marketing?
If you're relying on gut feelings to drive marketing decisions, you're likely leaving money on the table.
"First and foremost, guessing is bad," says Katie Robbert, CEO of Trust Insights, a data science firm. "If you're not using your own data to make the decision, you are likely introducing some sort of an unconscious bias."
Another common issue with traditional marketing is wasted resources, says Katie Robbert:
“Predictive forecasting tells you where to spend your money: when to step up, when to step down, when to spend money on ads, when not to spend money on ads. The worst possible thing you can do is just start throwing money at everything, and hoping that sticks, because that rarely ever works out.”
The numbers back this up. Companies using predictive marketing are seeing as much as 500% higher profitability by targeting the right customers with the right offers at the right time—something that's not possible with traditional marketing approaches.
Predictive marketing benefits include:
- Strategic decision-making: Use data analytics for smarter, faster, more strategic choices.
- Better lead quality: Identify your ideal customer profile with sophisticated segmentation.
- Meaningful personalization: Send targeted messages (and targeted offers) that are likely to inspire action in each user persona based on their behavior.
- Efficient resource allocation: Allocate marketing efforts based on predicted ROI.
- Improved revenue forecasting: Build more accurate forecasts by identifying buying signals and predicting customer lifetime value.
4 Common B2B Predictive Marketing Applications
There are endless ways to apply predictive marketing, but not all of them are worth your time. Here are four proven approaches.
1. Churn Prevention
No SaaS company is immune to churn, but the most successful ones see it coming before it happens. Take Hydrant, for example: by using predictive AI to spot at-risk accounts early, they achieved a 260% higher conversion rate and boosted revenue per customer by 310%.
The key is moving from reactive to proactive retention strategies. As Dr. Cristina Tran, a marketing consultant, explains, predictive analytics can:
"…help companies identify the underlying causes of customer churn and address them on a systemic level. By analyzing data on customer interactions, purchase history, demographics, and even data from social listening, companies can gain insights into which factors are most closely associated with churn. This can help them to identify specific areas where they need to improve, such as customer service, product quality, pricing, and sales interactions."
For example, let’s say you run a SaaS app and want to catch churn early. You can use predictive marketing to analyze customer data from various data sources, like CRM systems, usage metrics, and support interactions.
From there, you can design a retention campaign that segments customers by their propensity to churn. Like the example below, you’ll want to offer different messaging to each predictive segment:
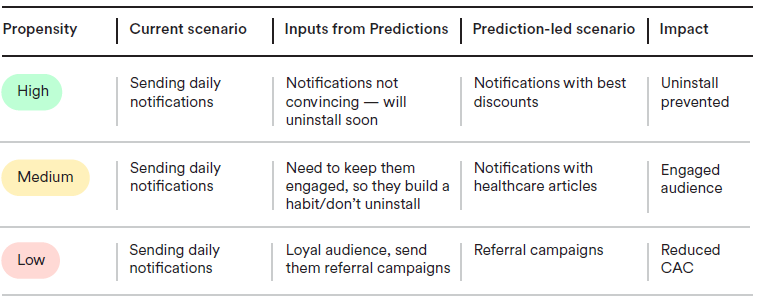
Source: moengage
Pro tips:
- Build a unified dashboard tracking product usage and customer health
- Set up automated interventions triggered by risk indicators
- Focus retention resources on the highest-risk accounts
2. Demand Forecasting
While predictive marketing isn’t a crystal ball, it can help you make crucial resource allocation decisions based on demand forecasting. "Predictive analytics is about understanding what will happen in the future based on historical data," explains Katie Robbert of Trust Insights. "It allows businesses to anticipate demand, optimize inventory, and align their resources accordingly."
By combining traditional data with real-time market signals and buyer intent data, you can forecast future outcomes with remarkable precision. For example, Statworx, a data science consultancy, developed a forecasting engine with the ability to estimate demand for thousands of products up to 24 months into the future. The model also increased the accuracy of demand forecasts by 10%.
Pro tips:
- Combine first-party data with market intelligence for a fuller context
- Develop multiple forecast scenarios (conservative, expected, aggressive)
- Set up regular forecast reviews with sales and product teams to validate predictions
3. Lead Scoring
Traditional lead scoring leaves money on the table. While basic demographic and firmographic data might tell you who your leads are, predictive lead scoring reveals which ones are actually ready to buy.
By analyzing thousands of data points, predictive lead scoring models can improve the quality of leads while helping your marketing team focus on the accounts that are most likely to close. For example, ActiveTrail, an email marketing provider, saw a 25% increase in opportunities and a 20% increase in deal closing rates after adopting a predictive lead scoring system.
Once you have a reliable lead scoring system in place, you can go a step further and design custom conversion strategies for each bucket of leads. For example, instead of offering the same incentives to everyone, you can offer different discounts based on each lead cohort’s propensity to convert.
Here's what this looks like in practice:
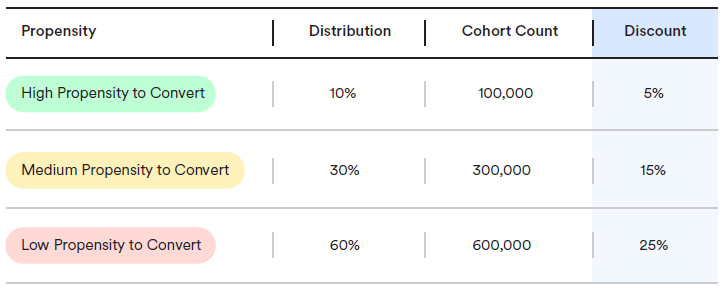
Source: moengage
Pro tips:
- Align scoring models with revenue impact, not just activity
- Layer behavioral and intent signals on top of basic demographic data
- Calibrate scoring systems using direct feedback from your sales team
4. Content Personalization
Generic content doesn't cut it anymore in B2B. With content personalization, you can use predictive analytics to understand exactly where each account is in their buying journey, what challenges they're facing, and what information they need next. Then, you can automatically deliver the right content through the right channel at the right moment.
For example, Gemini Sound, an ecommerce brand, uses personalized marketing to predict buyer intent based on real-time behavior (and based on similarities to current customers). Then, its content personalization engine serves up products that each visitor is most likely to be interested in. The result is a 73% increase in Gemini’s visitor conversion rate and a 128% boost in revenue per visitor.
Pro tips:
- Adjust messaging in real-time based on engagement signals
- Use machine learning to power smart content recommendations
- Run continuous experiments to optimize personalization strategies
Recommended Reading: 7 Predictive Analytics Examples To Inspire Your Next Move
B2B Predictive Marketing Best Practices
Predictive marketing success depends on four key pillars: robust data collection, compliant systems, continuous testing, and powerful analytics tools. Here's how to approach each one.
1. Collect Quality Data
Your predictive models are only as good as the data that powers them. That’s why your first goal when building a predictive marketing program should be a laser-tight focus on data quality. There’s an incredible amount of data you could be collecting, so you’ll need to hone in on the most important positive and negative signals.
On the positive side, you'll want to monitor regular interaction with marketing campaigns, frequent app or website visits, and product-specific searches. High-value behaviors also include consistent monthly activity, engaged responses to notifications, cart additions, and self-directed pricing research.
Just as important are the risk indicators that could signal trouble ahead. Watch for extended periods of inactivity, irregular usage patterns, and systematic notification dismissals. Other red flags include email and SMS disengagement, communication opt-outs, problematic support interactions, and negative feedback submissions.
Pro tips:
- Create systematic processes for data collection across all channels
- Pull in data from multiple sources, including CRM, social, and intent signals
- Balance historical patterns with real-time signals
2. Build Data Collection Systems that Comply with Data Regulations
Regulations like GDPR and CCPA have raised the stakes for data collection. The good news is that companies that comply get a double benefit: they avoid costly compliance issues while building trust with customers through transparent data practices. (This trust can also translate into better data quality since customers may become more willing to share information.)
The key to following data privacy regulations is to create clear consent mechanisms that explain exactly how customer data will be used. You’ll also need to design data governance frameworks that track and protect sensitive information and conduct regular audits.
Pro tips:
- Document every touchpoint where customer data is collected and stored
- Conduct regular compliance reviews and update processes as regulations evolve
- Implement granular data access controls and encryption
3. Institute a Test-and-Learn Approach
In his book Predictive Analytics, author Eric Siegel puts it bluntly:
"Once you develop a model, don't pat yourself on the back just yet. Predictions don't help unless you do something about them. They're just thoughts, just ideas... The key is to continually test and refine your models against real-world outcomes."
Even the most sophisticated predictive models can drift over time as market conditions change and customer behaviors evolve. That means a philosophy of “testing-and-learning” is necessary to keep your predictive marketing programs effective.
Start by regularly validating your prediction models against fresh data to ensure their accuracy hasn't degraded. You’ll also want to keep an eye on performance metrics that signal when your models need adjustment.
Pro tips:
- Schedule monthly algorithm validation checks against recent conversion data
- Track leading indicators that might signal model drift
- Set a regular cadence for model updates
4. Use the Best Analytics Tools
Your predictive marketing strategy is only as powerful as the tools you use to execute it. As you evaluate options, keep an eye on these critical factors:
- Seamless integration: Your predictive platform needs to pull data seamlessly from your CRM, marketing automation tools, and web analytics. Make sure it works flawlessly with your existing martech stack.
- Modeling capabilities: Make sure your analytics platform can analyze historical data, accurately forecast future behaviors, and create custom models and workflows.
- Enterprise-grade security and compliance: Choose tools with robust security measures and built-in compliance with GDPR, CCPA, and other data privacy regulations.
For in-depth tool recommendations, check out our list of the best marketing intelligence software.
Unlock Better Outcomes With Predictive Marketing
Predictive marketing isn't just about better forecasting—it's about driving better outcomes. To quote Katie Robbert one more time:
"The goal is not to predict; the goal is to change behavior to change outcomes. Predictive analytics is meant to guide you into the right direction to make a more data-driven decision than just guessing."
You can use predictive marketing to fundamentally transform how you engage with customers. By moving beyond “gut feelings” and using proactive, data-driven strategies, you can spark higher conversion rates, deeper customer relationships, and more efficient growth.
For more on predictive marketing, check out our guides to marketing software trends and prescriptive vs predictive analytics. And don’t forget to subscribe to The CMO Club newsletter to stay ahead of market trends and get more strategic insights designed for marketing leaders.